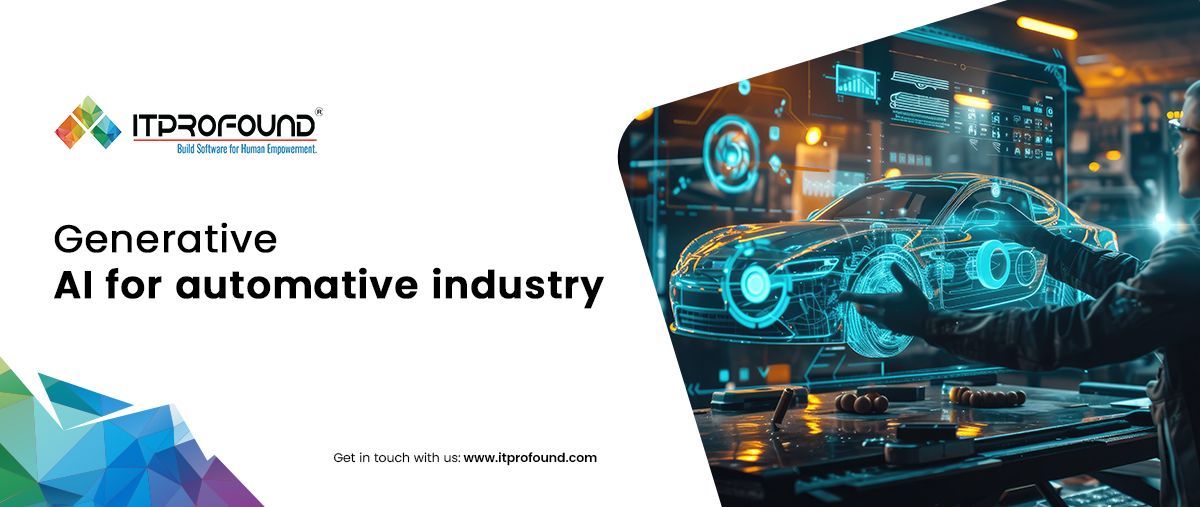
The Shift to Electric Vehicles (EVs)
Switching from gas-powered cars to electric vehicles (EVs) is a big change in car technology. This shift is like how the car industry had to respond to high oil prices over 50 years ago. Now, companies need to spend a lot on research and development (R&D) to create new power systems, better batteries, and the infrastructure needed for EVs. This means they have to rethink and change their usual R&D methods.
Electric vehicles are a major change from traditional gas-powered cars. This shift is as important as the car industry's reaction to past oil crises when they had to quickly make more fuel-efficient cars. Today, automakers need to invest heavily in new technologies for EVs, including better batteries, power systems, and the infrastructure to support them. This change requires car companies to rethink and redesign their R&D processes.
How Software is Shaping the Future of Modern Vehicles
Another big trend is the rise of software-defined vehicles. Modern cars now rely a lot on advanced software for features like entertainment systems and driver-assistance systems (ADAS). This shift means car companies need to focus more on software development instead of just hardware. The challenge is to smoothly integrate software into the car's main systems. Doing this well can help companies stand out in a crowded market. Those who succeed will have a big advantage.
The Rise of Generative AI
Generative AI is poised to significantly impact automotive research and development (R&D). While still in its early stages, generative AI has the ability to understand and process language and images, gather insights from various sources, and generate detailed documents. This technology holds the potential to transform the R&D landscape by streamlining and accelerating the development of new vehicles.
One of the key advantages of generative AI is its capacity to handle vast amounts of data and generate actionable insights. For automotive companies, this means they can more quickly and efficiently analyze market trends, customer preferences, and technical requirements. Generative AI can also assist in designing new vehicle features, improving safety systems, and optimizing performance by simulating various scenarios and outcomes.
New players in the automotive sector, especially electric vehicle (EV) manufacturers in China and the United States, are already leveraging generative AI to gain a competitive edge. By using this technology, they can speed up the time it takes to bring new vehicles to market, which is crucial in a rapidly evolving industry. This early adoption of generative AI provides these companies with a strategic advantage, allowing them to outpace traditional, established car manufacturers who are facing tighter profit margins and slower innovation cycles.
The Power of Generative AI in Car Research and Development
Top European car and manufacturing companies see a lot of promise in generative AI. Many are already trying out gen AI applications, with 75% of those surveyed experimenting with at least one, and the rest planning to start within a year. Companies are also putting significant money into this technology. Over 40% have spent up to €5 million on gen AI for research and development (R&D), and more than 10% have invested over €20 million. This shows that these companies are very confident that gen AI can change and improve their R&D processes.
Using Gen AI in Research and Development
Many companies are starting to use gen AI in their R&D processes, but most are only testing it in one part of their work. Still, they're exploring a wide range of uses, like requirements engineering, software testing, validation, and product design. This shows that companies plan to use gen AI more fully in the future. Executives believe that gen AI could improve R&D processes by 10 to 20 percent. In some cases, the benefits can be even greater. For example, a major German automotive supplier saw a 70 percent boost in productivity by using gen AI to generate test vectors.
Overcoming Implementation Barriers
Using gen AI in R&D has its challenges. To get the most out of gen AI, companies need to make significant organizational and cultural changes. It’s important to focus on the value gen AI can bring, clearly communicate its benefits, and have strong support from leadership. Engaging key internal groups, including those handling legal, ethical, and compliance issues, is also vital. Addressing concerns like data privacy and bias helps build trust and ensures everyone in the organization is on the same page, making gen AI easier to implement successfully.
Boosting Talent and Updating Work Models
Gen AI will act like a helper for employees, handling repetitive tasks so they can focus on more interesting work. To use gen AI effectively, companies need teams with experts from different areas, simple processes, and clear goals. It’s also important to have strong technology and good data management to support gen AI as it grows. Ensuring high-quality data and proper management is key to making gen AI work well.
Unlocking the Full Potential of Gen AI
To fully realize the benefits of gen AI, companies need a structured approach. They should carefully choose and prioritize the best applications for gen AI. After testing these applications, they should develop them into complete products with strong support for managing change and building capabilities. A clear vision and ongoing adjustments to the gen AI strategy will help companies quickly and effectively capture its value. This method ensures that gen AI innovations are successfully integrated into their R&D processes.
Conclusion
Generative AI is a game-changing tool for automotive R&D. By focusing on the value, it brings and overcoming organizational and cultural challenges, companies can gain substantial benefits. This includes cutting costs, speeding up innovation, and enhancing product quality. As the industry evolves, effectively integrating gen AI will be essential for staying competitive and fostering future growth.
Transform Your Business Now!
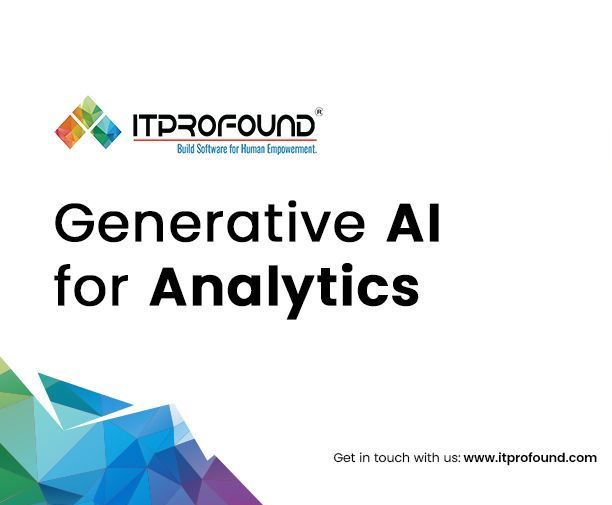
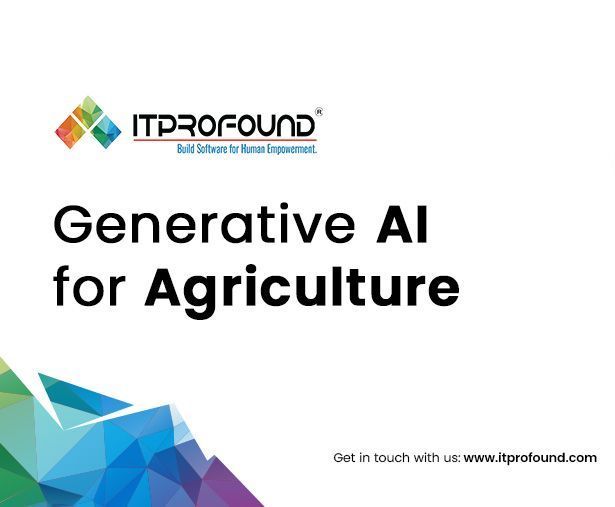
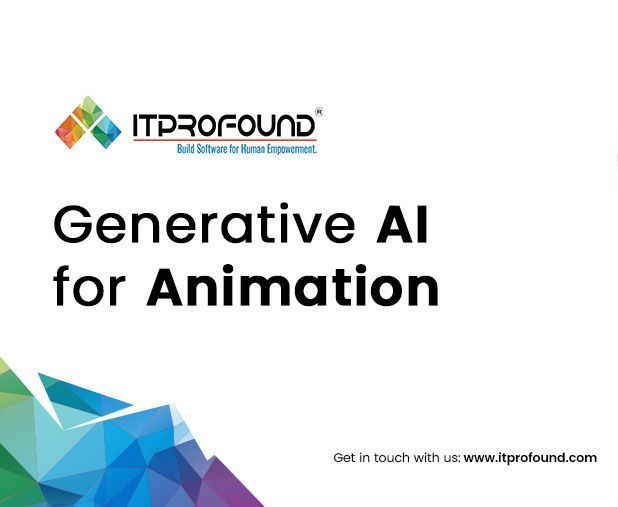
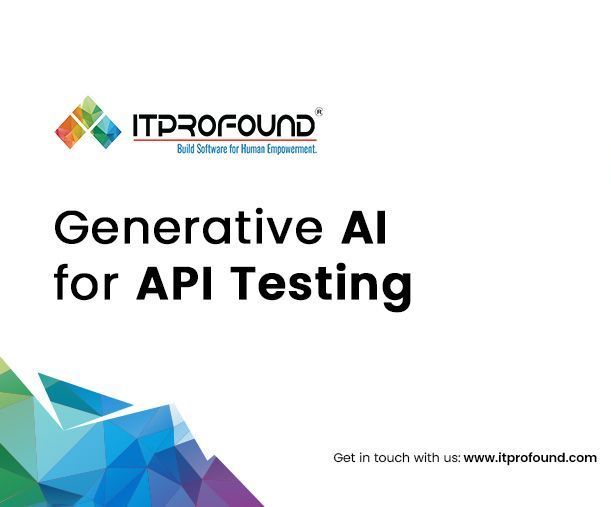
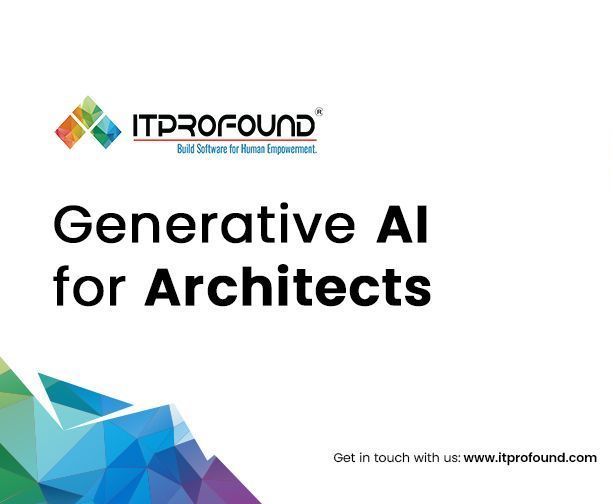
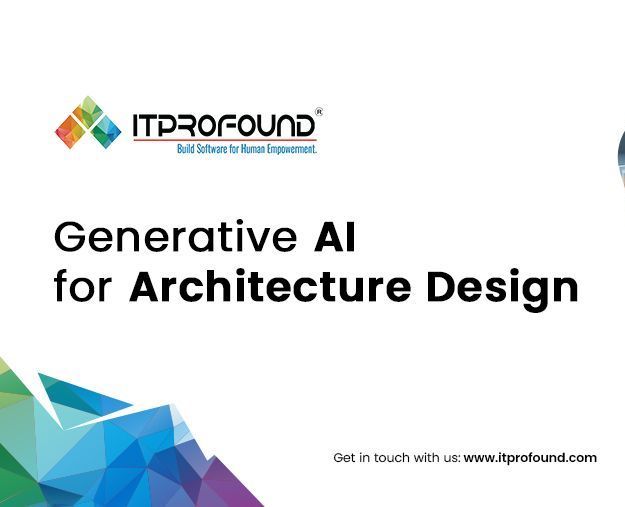
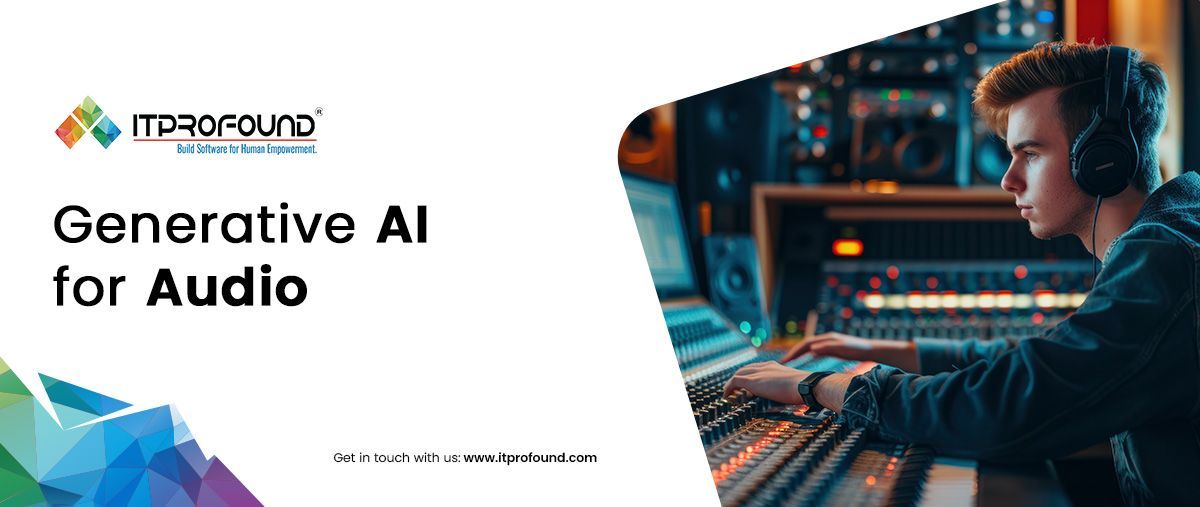
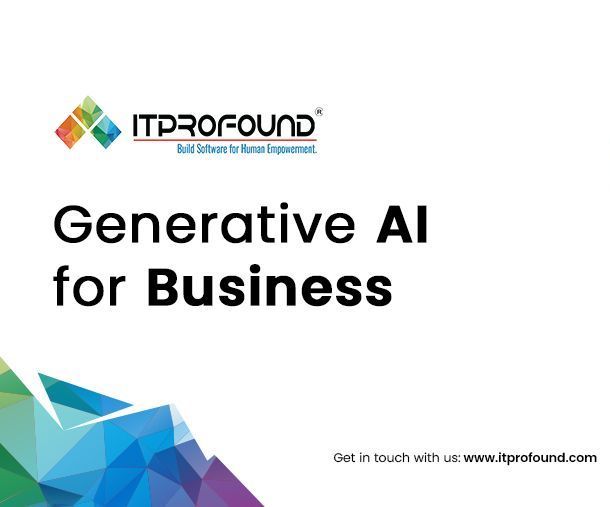
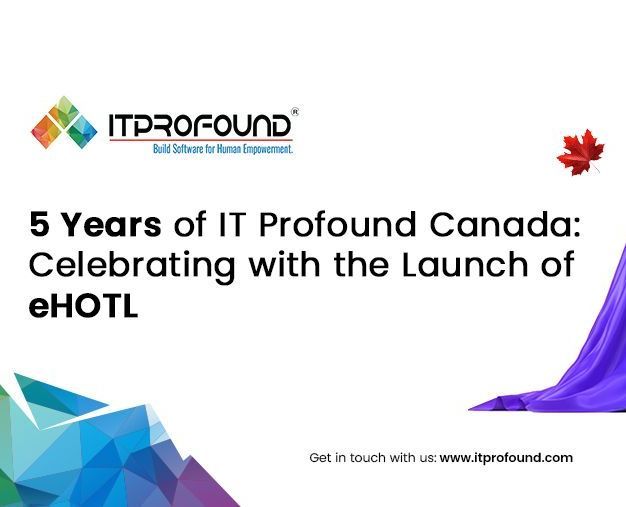